Supercharge Sales Operations with Generative AI
I’ve been using Generative AI aka Large Language Models (LLMs) to help me with day to day document preparation for over a year now and I’ve noticed that LLMs are really good at combining information about my business with existing information to produce customised and personalised documents.
This capability opens up some exciting opportunities for sales operations teams, to use AI to supercharge their document preparation process.
For example I have an existing proposal for AI Discovery Workshops that I have used for previous clients. I can feed this into the LLM in combination with information about a new client to produce an updated, personalised and relevant proposal for that new client. These AI outputs are astonishingly good quality and I’ve found it requires minimal time to refine this draft into a client ready document.
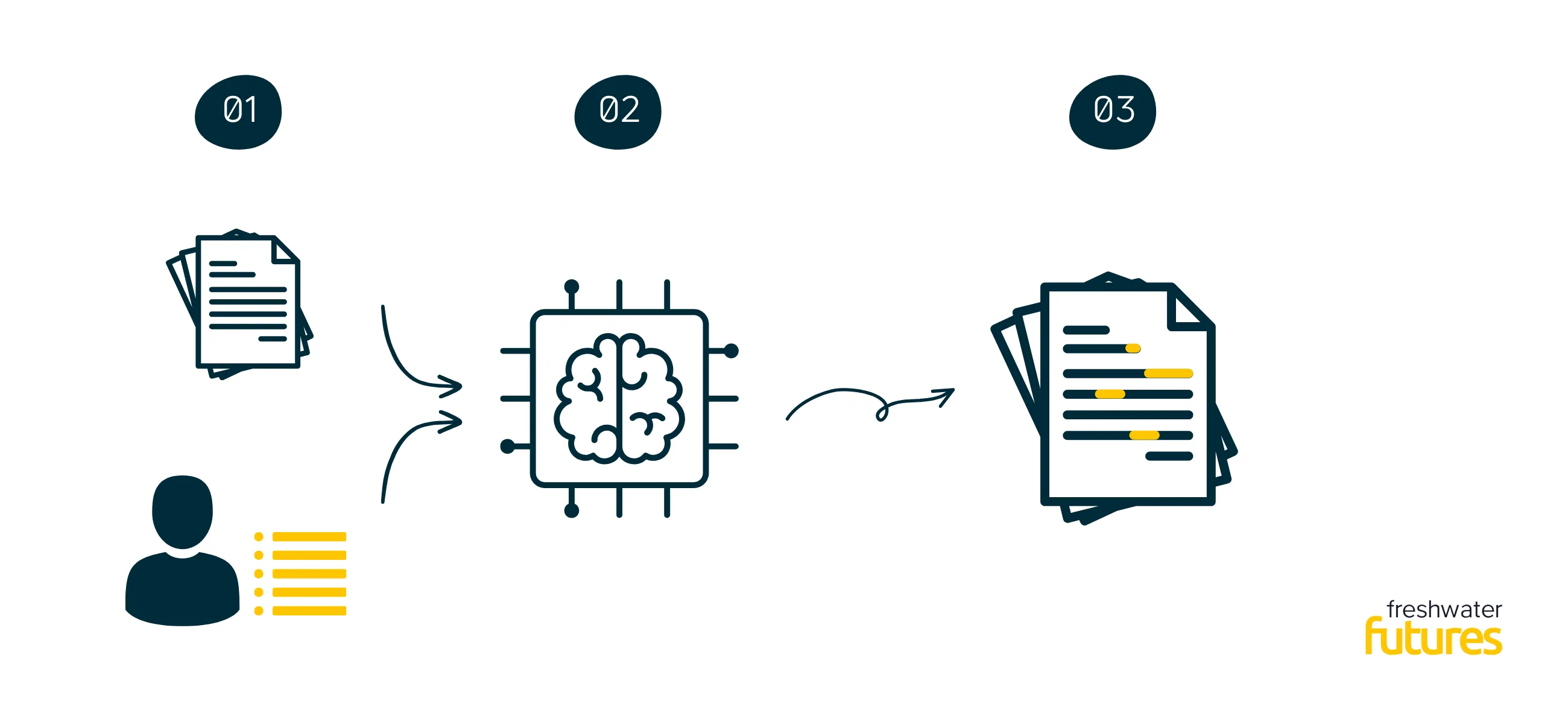
Challenges for Enterprise Sales Teams using AI
Many teams I talk to are yet to have access to ANY Generative AI at work. There are some very good reasons given for this and they boil down to three topics: security, cost and reliability.
Having spent over 20 years working with enterprise technology teams I’m very familiar with these types of constraints and recently I have been using this experience to help clients overcome these issues to unlock the productivity benefits of AI in a safe, cost-effective and reliable way.
Security and Privacy of Client Data
A primary concern for most CTOs is the risk of leaking sensitive client data to third-parties. This risk is much higher with Generative AI as the consumer-grade GenAI products (OpenAI’s ChatGPT, Google’s Gemini and Microsoft’s Copilot) have a very poor track record on data privacy and security. They go as far as to record and use your conversations to build future products when used with their default settings. Needless to say this is an unacceptable practice and data risk for any business.
In partnership with leading cloud vendors I’m developing secure, locally run and locally supported generative AI services running in local Australian data-centres. This means your client data never gets sent to Open AI, Google or the US. For international clients we use local data-centers so that your data does not leave your country.
In addition our approach provides legal assurances that your data is not stored or used by anyone beyond a 30-day safety period.
Typically we will work with our clients existing cloud vendor to take advantage of the existing legal data protection agreements.
We plan to extend our offerings to include AI privacy protection to automatically help teams proactively protect private client information.
Cost of Enterprise AI Products
The enterprise edition of ChatGPT (with SSO and other enterprise friendly security features) can be very hard to buy and is reportedly priced at up to US$60/user/month for a minimum of 150 users, so AUD$165,000 to start.
Microsoft’s enterprise edition of Copilot is US$30/user/month (minimum 1 year) so for a team of 10 that’s AUD$5,500/year. For their Copilot for Sales it’s US$50/month adding CRM integration (AUD$9,000 for 10 users per year).
Google’s enterprise edition of Gemini is also US$30/user/month.
One issue with the billing model of these products is you get charged every month even if a user does not use the AI.
I can also offer clients an alternative billing model: a pay-as-you-go usage model that allows you to share your AI budget across unlimited users and you won’t get charged for users that don’t use the service. This is also a great option for smaller teams looking to start with no upfront commitment while getting all the same protections of the enterprise products.
Reliability of AI outputs
Many early users of ChatGPT noticed it often made mistakes and produced inaccurate responses.
After many months of experimentation we now have a few powerful ways to address these issues:
- Use the most powerful model available: unlike early AI models the most powerful models today have vastly improved accuracy.
- Use a comprehensive automated testing approach: as underlying AI models are updated and when your needs change it’s important to be able to refine and update the underlying instructions to the AI. To prevent unexpected and unwanted responses from your AI we strongly recommend using a robust and disciplined approach to testing your AI.
- Use a fine-tuned model that has been customised and trained on relevant use cases. This is a more expensive option to setup, but can provide extremely accurate and fast responses and can even reduce running costs longer term. We recommend this step once the system has proven itself in your environment.
I offer clients a combination of all these approaches, typically over phases of discovery, prototyping and production.
The future of sales operations
Given we have ways to address three of the main obstacles to AI adoption for sales teams I see a future where most sales teams will start using AI as a core part of the sales operations process.
Would you like a demonstration?
To demonstrate the power of this technique I’ve built a simple application that asks for information about a client (industry, pain points, company name etc) and the combines that with a proposal template to generate a new personalised document. Book a call below to get a demo and to chat about your use case.